Mathematics News
Former WKU Graduate Student Proposes Breakthrough in Robust Matrix Completion through Deep Learning: Virtual Talk on February 14, 2024
- Zulfiqur Haider
- Thursday, February 1st, 2024
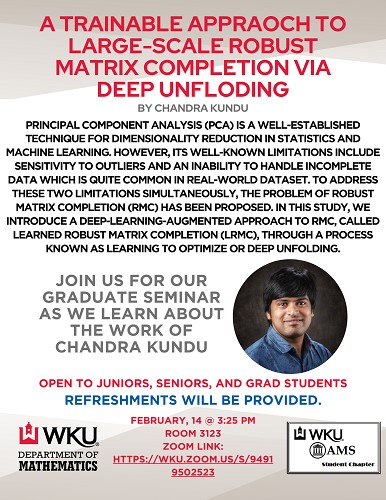
In an upcoming virtual talk on February 14, 2024, Chandra Kundu, a former graduate student of Dr. Lukun Zheng at Western Kentucky University (WKU), now a Ph.D. candidate in Big Data Analytics at the University of Central Florida, will unveil a groundbreaking approach to address the limitations of Principal Component Analysis (PCA). His talk focuses on a cutting-edge technique known as Learned Robust Matrix Completion (LRMC), designed to overcome PCA's well-known challenges related to outliers and incomplete data.
Principal Component Analysis has long been a staple in statistics and machine learning for dimensionality reduction. However, its Achilles' heel lies in its sensitivity to outliers and its inability to handle the prevalent issue of incomplete data in real-world datasets.
Enter Robust Matrix Completion (RMC), a proposed solution that tackles both challenges simultaneously. Kundu's LRMC takes RMC a step further by incorporating a deep-learning-augmented approach through a process known as Learning to Optimize or Deep Unfolding.
The method of Deep Unfolding involves unrolling a conventional iterative algorithm into a deep neural network (DNN), where each iteration becomes a layer of the network. This innovative approach transforms the hyperparameters of the original algorithm into trainable parameters (weights) of the DNN. By doing so, Kundu's LRMC optimizes the performance of the proposed algorithm for a specific class of RMC problems through the techniques of DNN training.
Extensive empirical experiments on synthetic datasets and real-world applications underscore the potential of LRMC, showcasing its superiority over state-of-the-art methods. The results suggest that LRMC presents itself as an attractive choice for addressing PCA problems burdened by both outliers and missing entries.
Chandra Kundu's virtual talk on February 14 promises to shed light on this transformative approach, providing insights into the realm of deep learning applications in robust matrix completion. As a former WKU graduate student, Kundu's pioneering work exemplifies the continued impact of WKU's academic community on cutting-edge research in emerging fields. The WKU community eagerly anticipates the revelations from Kundu's presentation and the potential implications for advancing data analytics methodologies.
Link to the Virtual Event: https://wku.zoom.us/j/94919502523